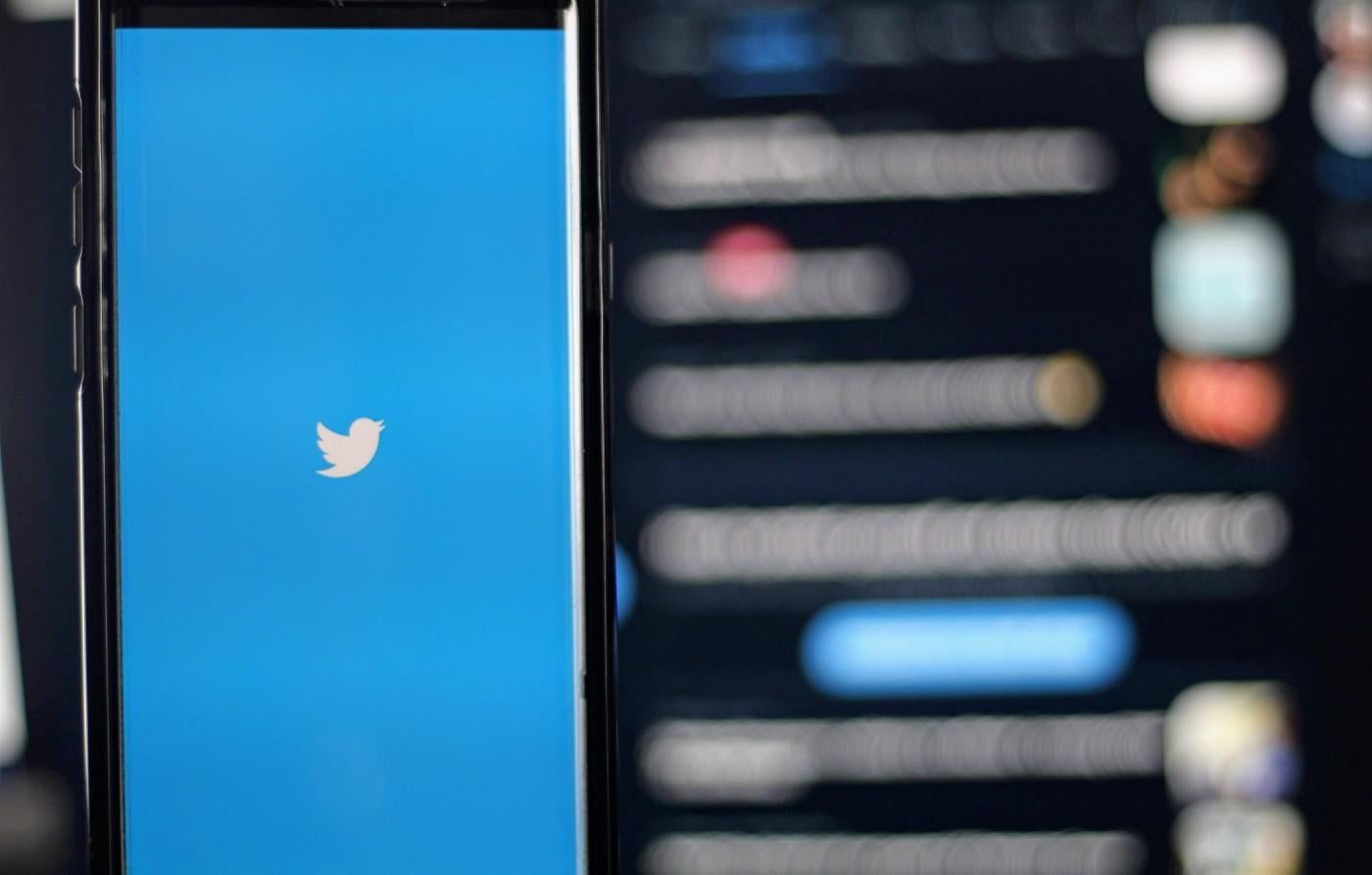
After Hurricane Sandy devastated parts of the East Coast in 2012, 911 systems became so overloaded that people couldn’t get through by phone to report an emergency. At a loss for how to reach out for help, people turned to Twitter to post distress calls, which the New York Fire Department began fielding and responding to.
It was one of the first times social media was used this way during a major disaster, demonstrating that the internet can be a powerful tool when it comes to emergency response.
But with millions of people posting content on platforms like Twitter and Facebook every second, it’s nearly impossible for a human person to sift through the mountains of data to find the important information that could help during events like fires, floods, and other disasters.
That’s where machines come in.
Through machine learning, computers are able to recognize patterns in data, which allow them to scan the myriad of social media posts and find key content that could alert emergency managers to dangerous situations — words like “help,” “trapped,” and “stranded.”
But they can’t do it on their own. Someone has to train the computers to recognize what’s critical information and what isn’t. You can tell a computer to search for keywords like “flood” and “pandemic,” but in addition to getting some potentially crucial information, you’ll also get a bunch of content that might not be helpful at all — political statements, funny memes, and even advertising. (People often take advantage of trending hashtags during emergencies just to get eyeballs on their content.) Someone has to help the computers weed out all that noise.
That’s where a multidisciplinary team from The University of Texas at Austin, Brigham Young University, George Mason University and Virginia Tech comes in.
A case study in disaster response
Funded by a National Science Foundation grant, they have been collaborating with a Community Emergency Response Team (CERT) in Maryland, interviewing volunteers as they labeled social media data to help computers learn to identify disaster-related content. They monitored as the volunteers reviewed the computers’ work and decided whether the information it flagged was relevant or not. Their work helps refine the algorithms so that the computer becomes more efficient over time. The hope is that someday — perhaps many years down the road — computers will be able to effectively do much of this work on their own with limited human intervention, which could be a major benefit to emergency managers.
“We live in this interesting time where the public wants to take advantage of the fact that we have all these social media outlets we can post in, but the emergency management community hasn’t kept pace,” says Keri Stephens, a professor in Moody College of Communication’s Department of Organizational Communication Technology, who is leading the project. “By training these machines, we can help them catch up.”
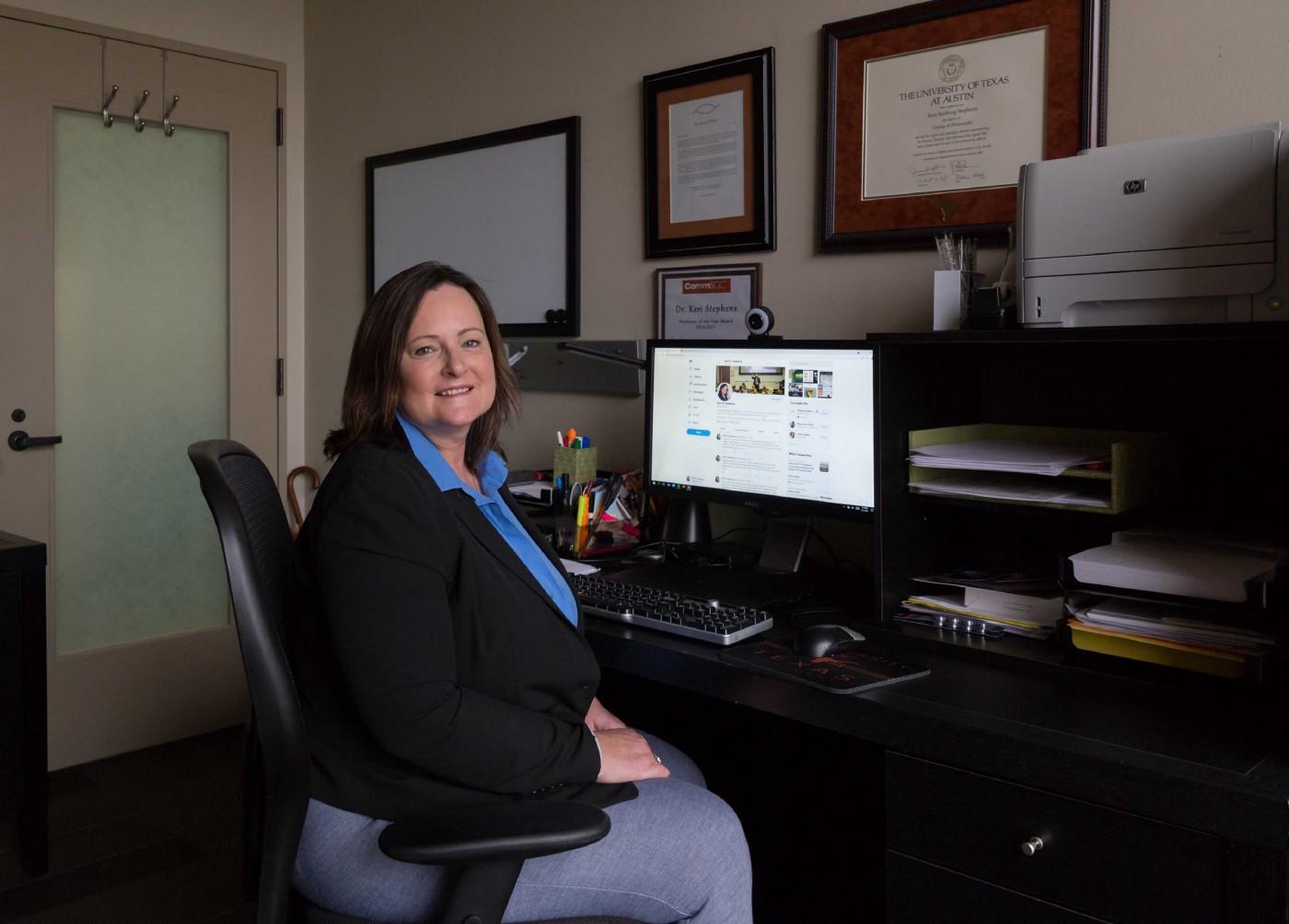
The team started its work last summer using the COVID-19 pandemic as a case study, looking for incidents like people not wearing masks or social distancing. They focused specifically on the Washington D.C. area, using a computer system called “Citizen Helper.” The computer starts the process by scanning Twitter for keywords, then the volunteers review the tweets in chunks of 500 to refine the computer’s algorithm.
“It really is a human-machine interaction,” says Stephens. “The computer is trying to categorize things, but it doesn’t know what it’s looking at. The humans teach it what is a risk so the next time it recognizes similar social media data as a risk.”
As volunteers scrutinized the data, researchers conducted observational interviews, taking notes as they tried to understand why the volunteers labeled certain posts as relevant.
“There’s a lot of subtle information about how to interpret data,” says Amanda Hughes, a professor in Information Technology and Cybersecurity at Brigham Young University. “A lot of times, the volunteers bring in information from their background that helps them understand what is going on in an emergency. And there is a lot of value to being local to the area where the event is happening. You are able to pick up on things that someone from a different area wouldn’t understand, such as the history behind a local landmark or a politician. That kind of contextual knowledge is something that’s really hard to build into AI. It’s going to take many years to get to the point where we can detect those kinds of things, but small steps in that direction allow us to understand the process by which people make those decisions.”
One of the goals of the project is to minimize the need for human intervention in the algorithm, so that the computer can clearly define what COVID-19 prevention and risk looks like on its own, says Steve Peterson, a certified emergency manager and community coordinator for the project. This would allow emergency managers to more easily respond to areas where large groups of people are gathered without masks or provide more staff at vaccine sites to help maintain social distancing.
Peterson says a similar process could be used to help with all sorts of disasters, including hurricanes, fires and windstorms.
“Technology is not the priority of public safety. The public’s safety is the priority. I think that’s one of the biggest challenges is trying to convince emergency managers that the data that can be generated from a machine can be used with great confidence because it’s been developed by trained, credentialed volunteers.”
With the new knowledge obtained from the project, CERT volunteers, who are affiliated with the Federal Emergency Management Administration, could drop in anywhere in the country and rapidly train computers to recognize important information, which would help emergency managers respond to dangerous situations, including rescuing people from floodwaters or carrying out evacuations during a wildfire.
“There’s a lot of garbage on social media, but there’s also a lot of useful information that could be helpful to first responders,” Hughes says. “We are trying to provide the tools to help that process so that social media can be a more trusted information source.”
Creating a robust curriculum
The research team recently applied for a $1 million NSF grant and hopes to receive funding that could be used to update the training curriculum for CERT volunteers so that, in addition to the current work they do like search and rescue and first aid, they can also help to train computer algorithms to spot disaster information. FEMA’s current curriculum hasn’t been updated in 20 years, Stephens adds — a lifetime when it comes to technology advances.
“This is an incredible way to take what we have learned and make it really practical, to create a robust training curriculum to help these volunteers understand what they are looking at when they look at Twitter data,” Stephens says. “It can help them make better decisions. The better their decisions, the faster we can train the machines.”
Currently, computer systems like Citizen Helper and other AI algorithms are not being used by many emergency managers in this context in the U.S. There’s not even a proprietary system they could purchase right now if they wanted to, Peterson says. But he hopes to change that by helping the emergency management community overcome its hesitancy with using social media, which is fraught with misinformation.
“Technology is not the priority of public safety. The public’s safety is the priority,” Peterson emphasizes. “I think that’s one of the biggest challenges is trying to convince emergency managers that the data that can be generated from a machine can be used with great confidence because it’s been developed by trained, credentialed volunteers.”