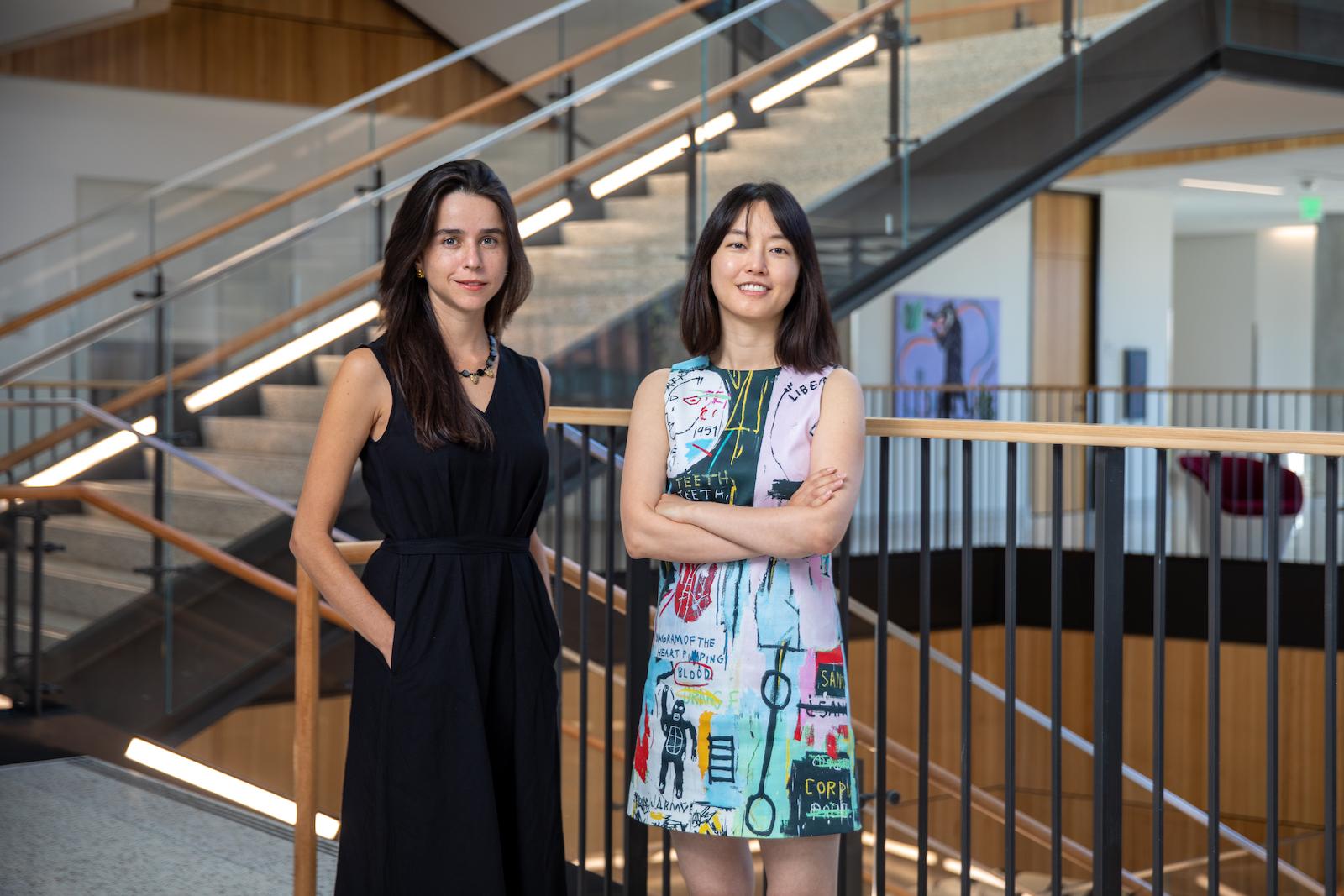
A Q&A with Maria De-Arteaga and Min Kyung Lee
In the past decade, machine learning — using machines to detect patterns in data and make decisions with minimal human supervision — has become a critical component in a variety of applications from healthcare to autonomous vehicles. But as artificial intelligence like this expands into more areas of our lives, it’s important to ask tough questions about who it’s serving, who it’s hurting, and how to mitigate the harms. We spoke with Information, Risk and Operation Management Assistant Professor Maria De-Arteaga and School of Information Assistant Professor Min Kyung Lee about some of these challenges — from the use of machine learning for predictive policing to the reliance on mobile apps for everything from transportation to smart homes — and how they address them in their work with Good Systems.
Tell me about your work and what drew you to your current research.
Maria: I’ve had a winding path to the work that I do. I completed my undergrad in math and then made my way to investigative journalism. I wanted to do data journalism, but things shifted, and I ended up getting a Ph.D. in machine learning and public policy. My initial focus was looking at how machine learning could be useful in the context of public policy, such as in providing health care and social services. Today, I am interested in the risks and opportunities of using machine learning systems to support expert decisions, such as how physicians make treatment decisions or how social workers intervene after a report to a child maltreatment hotline. The risks I consider include those associated with algorithmic fairness and the algorithmic harms that may result from the gap between algorithmic predictions and algorithmic-informed decisions.
For example, an algorithm meant to assist in targeted job recruiting may compound gender imbalances across occupations. It may also rely on stereotypes to make predictions, replicating and amplifying patterns of workplace discrimination. My hope is to bridge the gap between what we want to do with machine learning and what we are actually doing. This involves both designing novel machine learning algorithms, reimagining how we integrate machine learning in decision-making pipelines and understanding when we should not be using machine learning systems.
Min: My path was also very winding. I am a human computer interaction researcher in UT’s School of Information. I was always interested in machine agency, or the concept that machines can make autonomous decisions, and how we can make autonomous machines more human-centered. When I first started research as a master’s student, I was studying smart homes and exploring the appropriate level of machine automation that still respected human agency and control. My Ph.D. focused on robots, which still involves autonomy but instead of being embedded in the environment, it was embodying a physical body. I then got interested in the broader use of AI when working with Uber and Lyft drivers in 2014. These apps were like a robot performing work, but the difference was that it doesn’t have a body, it’s embedded in an app. I was curious about how people worked with this app — a robotic manager — instead of a human dispatcher like with traditional taxi services.
With apps like Uber and Lyft, they may seem benign, but they are really making a big impact on people’s lives because they determine people’s pay and income and how drivers are getting hired and fired. This became the seed for my current research, where I examine the effect of AI on civic issues and the workplace and explore ways to make AI embody important values such as fairness, equity, and well-being.
How is your work similar?
Min: We are both trying to improve AI decision-making. I think our ultimate goals are the same. Maria focuses more on the algorithm, while I focus on the constraints of the algorithm and how it should communicate decisions. For example, in my work, I try to quantify people’s concepts of fairness so that we can design algorithmic constraints or draw from procedural justice literature to design algorithmic transparency.
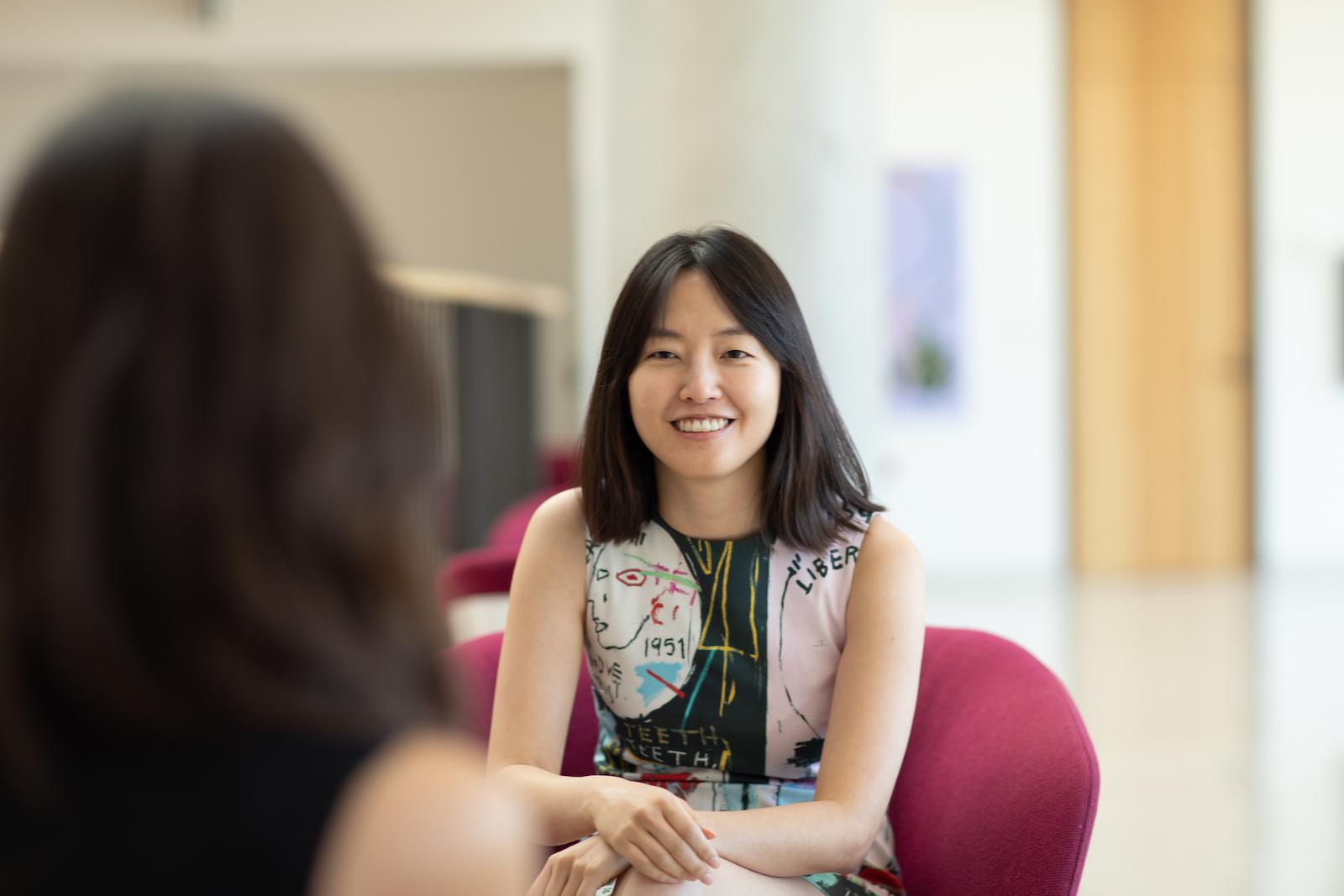
“We are not just doing research for academic publications. It’s important for me to remember that I am doing this work for myself and for the academic community but also for the community I am studying. I ask myself how I can make sure that the outcomes are designed to serve both.”
— Min Kyung Lee, Ph.D.
Maria: I would say that in addition to our end goals being the same, I think that the subject of what we study and are curious about is also very similar. But how we go about getting answers to these questions and proposing solutions are different. I love how Min said it: we use different materials. The tools that we each use and the lens that we think about things through are different. I think that’s what makes it very fun for me to collaborate and chat and learn from Min’s work. It’s very complementary.
How has the lens you’ve used in your work influenced your own personal lens? And how have you learned from each other’s work?
Maria: Over the past years, my research has evolved. The focus of my studies is still the algorithm, but now I’m considering how it interacts with people and how people interact with the algorithm. I think that being exposed to research like Min’s has really shaped the questions I’ve asked and the types of solutions I propose. I’ve realized there is no way to think about algorithms that are going to be used by humans without taking very seriously how humans are making use of these systems.
Min: Like Maria’s, I think my work nowadays also tries to give the end user more control over the algorithm itself, rather than assuming it is something the user cannot control. For example, we have been very interested in how we can empower people who are affected by AI to help design it, even if they are not experts.
For some reason, the way we do things today is to build everything and include people and users at the end of the process, rather than involve them upfront to guide decisions that direct the final product. If we involve them in the actual design, it is more empowering. And specifically acknowledging their participation is also very important. They are our co-collaborators, not just people whose opinions we ask so that we can design their things. We are also cognizant that any participation can be a burden on users. In this sense, people — especially those who are marginalized — may not be able to participate because they don’t have time. There is a risk of overburdening someone who is already overburdened, but there are ways to make participation easier, and that is what we are aiming for.
Maria: I want to echo Min’s emphasis on the risk of overburdening a community we are trying to serve. Concerns of overburdening or underserving some populations are what led me to shift the focus of my research during my PhD. For example, in one project we analyzed patterns of sexual violence in El Salvador. While our approach enabled us to identify important patterns that guided a journalistic investigation, using such a tool for something like allocating sex education resources to reduce domestic violence would introduce a risk of underserving some communities based on the data, since some women often underreport violence because they don’t trust institutions. You run the risk of not providing adequate resources to historically marginalized communities because they aren’t reflected in the data.
“While we often focus the conversation on self-accountability, this often neglects the power that communities have to hold institutions accountable and the work that they have done to achieve this.”
— Maria De-Arteaga, Ph.D.
Maria, you gave an example of survivors of domestic violence and how data did not reflect reality because some people didn’t report or didn’t trust institutions. How do you account for that in your work?
Maria: I think that a lot of my work in this space has been in questioning whether the machine learning tools that are proposed to address disparities are actually going to achieve that, precisely because, as you said, the data are often another vehicle for reproducing the types of biases we are concerned about. There is also a risk of compounding them and amplifying them.
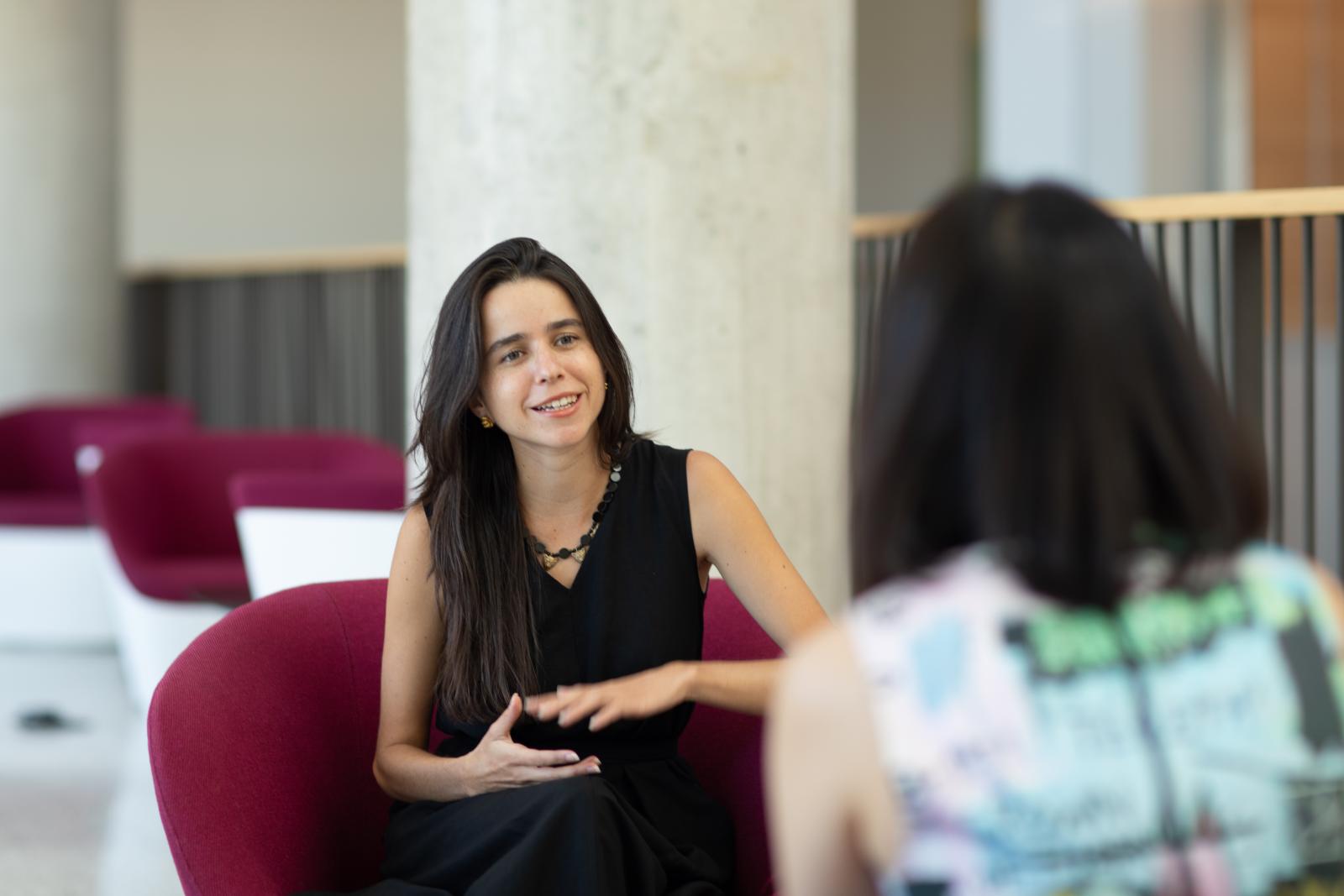
One of the areas where this is happening is in predictive policing. Research has shown that by looking at arrest data to determine who and what areas need policing, you are reproducing biases that have existed over time. So instead, people have shifted to using victim reports and 911 calls instead. My collaborators and I have looked at what happens when you train these systems with victim report data. My team took data from Bogota, Colombia that looked at whether people had been victims of a crime and if they had reported it. That mismatch allowed us to see what would happen when you are guiding predictive policing tools on crimes that were reported. For some regions in the city, you needed twice as much crime to lead to the allocation of police for that area because of the disparities in reporting. At the end of the day, I do not think there is always a solution to address this bias, and sometimes the answer is that there are systems that we should not build or deploy.
Min: We have also been thinking about the role of trust in human systems. People nowadays say they trust AI decision-makers less than human decision-makers. One way to mitigate this problem is what we call human-in-the-loop, where humans oversee AI decision-making. People trust AI when there is a human decision-maker overseeing it. But I’ve found that’s not always the case. When I was doing field work, we went to a food pantry and asked people what they thought about AI allocating the food. Initially I thought they would have a bad reaction. But they actually thought it wasn’t a bad idea because humans are so discriminatory maybe they thought it would be better. They said, “It wouldn’t see my skin color; I’d be OK with that.”
"The relationship between UT and the City of Austin, through Good Systems, allows us the opportunity to try something novel for the scholarly community and also for the community itself."
— Min Kyung Lee, Ph.D.
I have explored this question similarly in the context of healthcare. In another study, we found that Black communities were less trusting of AI in the healthcare system, even when overseen by doctors. Historically, they have more mistrust in these institutions, so if we say that human-in-the-loop is the right way, people who don’t trust doctors now won’t trust them even when operating AI. We have to find a way to account for this mistrust when designing AI systems.
What obligation do you have as researchers to be critical of your own pursuits?
Maria: In the machine learning community, for quite a few years, we were driven a lot by the “move fast and break things” culture of Silicon Valley, and I do think that spills over in general to how the machine learning community was pursuing things. The now-famous Facebook motto captures how tech companies were churning out products as fast as they could without considering their merit or rationale. Part of the awareness around algorithmic bias and harms has led us to have a reckoning of the way things had been done for many years. I think the hardest part for us machine learning researchers is knowing when machine learning is not the answer, and which things should not be built. I do want to note, however, that while we often focus the conversation on self-accountability, this often neglects the power that communities have to hold institutions accountable and the work that they have done to achieve this. I think that is what we have seen over the past years, which makes me hopeful. There are people literally protesting algorithms in the streets. That “move fast and break things” mantra is really changing because communities are holding the machine learning community accountable.
Min: When I started at UT and began engaging with Austin residents, they told me that, when they were young, researchers would come into the streets and neighborhoods, take pictures of them, conduct research, and then would just disappear. To them, researchers are just people who do research with them, and there is no return. They don’t know what happened with the findings. My hope is to make that interaction more bi-directional. We are not just doing research for academic publications. It’s important for me to remember that I am doing this work for myself and for the academic community but also for the community I am studying. I ask myself how I can make sure that the outcomes are designed to serve both.
What do you hope Good Systems will be able to deliver?
Maria: One thing that I am very excited about that is at the heart of what we have been discussing today is the need for interdisciplinary and multidisciplinary collaboration. That is something that is sometimes hard to achieve in academia because we are so siloed. This interdisciplinary approach is especially important when we have questions about emerging technologies, and the tools we need to answer these questions do not necessarily fit nicely in the way we have organized academic institutions. Good Systems really enables these multidisciplinary and interdisciplinary teams to come together to study these questions. Any university can say they support interdisciplinary work, but it takes so much to build an ecosystem where that kind of work exists, and Good Systems is that kind of ecosystem.
Min: I would like to echo what Maria said. It is not just social science, engineering, or computer science. In Good Systems, there is also deep involvement of the humanities, which I haven’t frequently seen in other interdisciplinary collaborations for AI research. I think Good Systems has the potential to deliver the UT Austin slogan: what starts here changes the world. Austin is a unique place. It is such a fast-changing city with a lot of tech initiatives, but I think the city size allows us to be nimbler compared to New York and other major cities. The relationship between UT and the City of Austin, through Good Systems, allows us the opportunity to try something novel for the scholarly community and also for the community itself. Good Systems was one of the main factors that motivated me to come to UT, so I have very high hopes for it.
Maria De-Arteaga Gonzalez, Ph.D., is an assistant professor in the Information, Risk, and Operations Management Department in the McCombs School of Business whose research focus includes using machine learning to support decision-making in high-stakes settings. She helped to found the ML4D workshop, which brings together researchers globally to use machine learning to solve problems in the developing world.
Min Kyung Lee, Ph.D., is an iSchool assistant professor. Lee has conducted some of the first studies that empirically examine the social implications of algorithms’ emerging roles in management and governance in society, looking at the impacts of algorithmic management on workers as well as public perceptions of algorithmic fairness. She has proposed a participatory framework that empowers community members to design matching algorithms for their own communities. Her current research on human-centered AI is inspired by and complements her previous work on social robots for long-term interaction, seamless human-robot handovers, and telepresence robots.