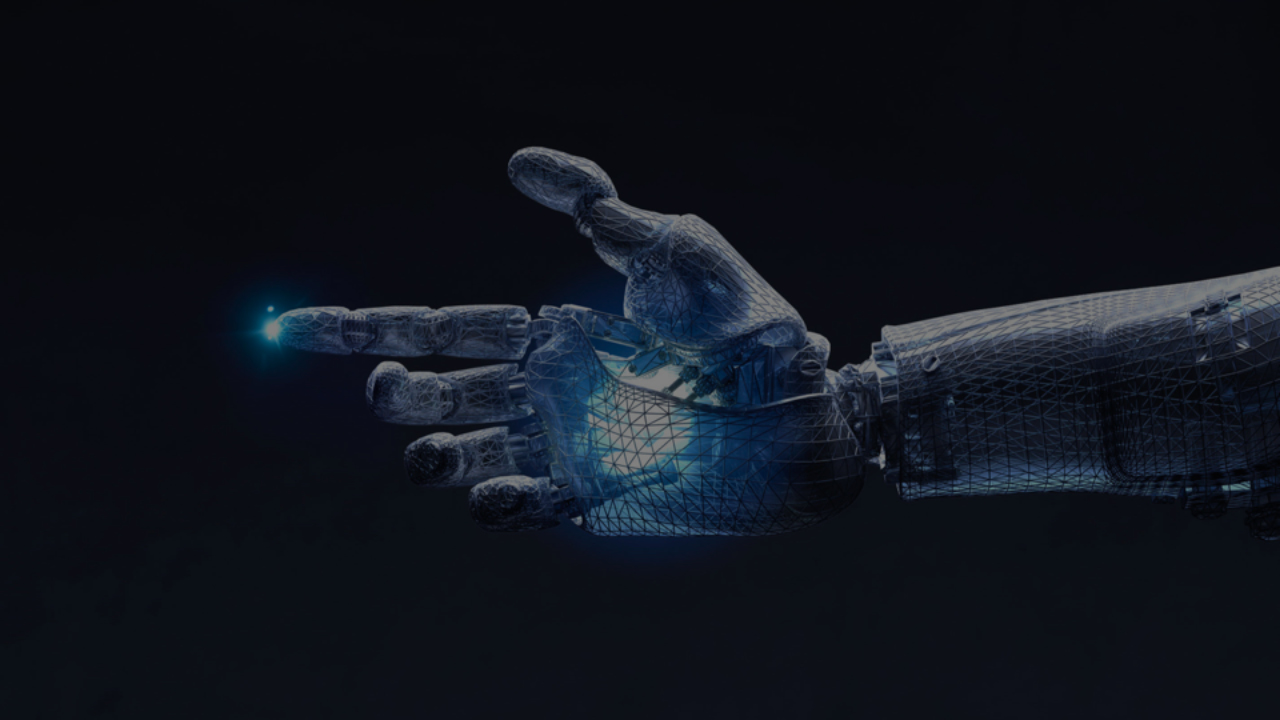
Artificial Intelligence (AI) improves our everyday lives. AI could end civilization as we know it. AI will create new jobs. AI will cause job losses. AI is revolutionizing transportation. AI causes aviation disasters. AI can help level the playing field in society. AI can exacerbate existing inequalities and create new ones. AI is already in a large number of devices throughout the modern home and can lead to significant efficiencies that can improve our quality of life. AI can spy on us and violate our personal privacy.
These contradictory facts illustrate Kranzberg’s First Law of Technology: “Technology is neither good nor bad; nor is it neutral.” Technology must be evaluated based on how it is used, by whom, for what purpose — and also on how it is designed, by whom, and for what purpose.
Our goal as a grand challenge is to avoid the dangers of carelessly experimenting with new technology. As a famous (albeit fictional) University of Texas at Austin mathematician said about those responsible for Jurassic Park: “Your scientists were so preoccupied with whether or not they could, they didn’t stop to think if they should.”
The Good Systems team here at UT aims to help technologists stop to think about what they are doing. We’ve embarked on an 8-year mission to design AI technologies that are driven by human values and that benefit society. We’ve done so because we believe it is ethically irresponsible to think about AI only in terms of what it can do; we believe it is even more important to consider what AI should — and should not — do.
AI technologies can be helpful and can improve the ways we live and work. They already do that every day. But problems arise when we fail to predict the potentially dangerous unintended consequences that can go along with AI technologies (privacy and data breaches, aviation accidents, unemployment, undermined elections, just to name a few). It is critical for us to be proactive instead of waiting to see what happens.
If we wait to see what happens and then attempt to regulate dangerous technologies after it’s too late, the results will likely be as effective as shutting the barn door after the horse is already out.
The “best solution to a problem” in a technical sense isn’t necessarily “good” for people.
What Is a “Good System”?
To answer that, we first have to know what we mean by “AI technologies.” Artificially intelligent technologies are systems that can correctly interpret data, learn from data, and use the insights they glean to identify the best solution to a problem. Yet the “best solution to a problem” in a technical sense isn’t necessarily “good” for people. In this sense, “best” typically refers to a measurement of accuracy, precision, and/or efficiency; it does not necessarily encompass the intentions or the impacts of the technology.
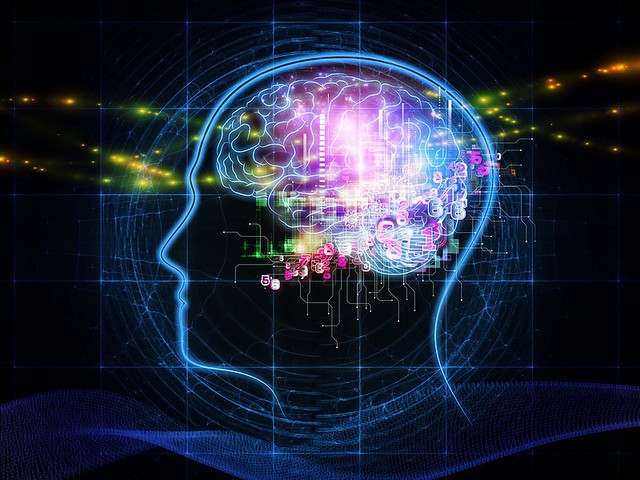
Think of AI as akin to an operation that can be a success even if the patient dies. Just as value-based care involves focusing on outcomes rather than performing operations for the sake of doing operations, the design and evaluation of AI technology should be “good” — not just on its own terms but also insofar as it benefits society. And that’s what we mean by “good systems”: they are human-AI partnerships that address society’s needs and values.
Of course, such a notion of “good” is not simple or straightforward. Philosophers and ethicists still debate what it means for a person to be good as well as how to evaluate the goodness of a person’s behavior. They have developed virtue and deontological perspectives that focus on intentions (even though “the road to hell is paved with good intentions”) and utilitarian perspectives that focus on consequences (even though “the ends don’t justify the means”). We have thousands of years of arguments about what it means for a person to be “good” on which to build as we consider how to ensure that AI technologies are good — which is a good thing since we don’t have thousands of years to figure this out!
It’s Not (Just) Killer Robots
AI isn’t just about killer robots. AI is in a huge range of products and services that many of us use daily. Not only is AI the potential future of driving, but it also helps us to drive more safely today. AI helps detect fraud and prevent identity theft, and — perhaps even more important — it also picks our next song on YouTube.
But even industry leaders fail to appreciate the role (and presence) of AI in their businesses: “While only about a quarter of surveyed business executives say they’re currently using artificial intelligence in the workplace to automate manual tasks, a vast majority of those who said they weren’t using AI actually were without realizing it.” As AI becomes increasingly self-aware and ever-present, it’s imperative that we become aware of the limitations of the AI technologies we’re using, that we understand how we’re using them and what the consequences could be, and that we all fully comprehend what they do.
That’s what we mean by “good systems”: they are human-AI partnerships that address society’s needs and values.
Most of us are on autopilot, assuming the apps and technologies we use are benign. The truth is that they’re not benign — but the dangers they pose weren’t usually intended by their developers. Rather, those dangers arise because developers themselves are often on autopilot; they unquestioningly follow routines they learned. They see everyone else following those routines as well, which reinforces their habits and choices. Langdon Winner terms this tendency “technological somnambulism” — sleepwalking through technology development.
When I teach ethics of AI, my goal is not to turn evil students into good students. (Students, in my experience, already tend to be generally good people already, especially students who voluntarily choose to take an elective ethics course. Moreover, moral character and values are most heavily influenced during childhood, and, as such, it is unlikely that one college course could significantly intervene to improve them.)
Instead, my goal is to get students to think about the ethical decisions involved in the design of AI-based technologies, not just the technical ones. (If there even are purely technical decisions. I would argue that there are not, because everything we do connects with our values.)
Empowering students to see design decisions as ethical decisions is the first step toward ensuring they know how to consider if their designs benefit individual stakeholders and society as a whole.
A Values-Driven AI Framework
What are human values? They can be understood as the “guiding principles of what people consider important in life.” Some examples include freedom, honesty, creativity, equality, and wealth. Since Aristotle, we have often thought of values as in tension, or in balance, with each other.
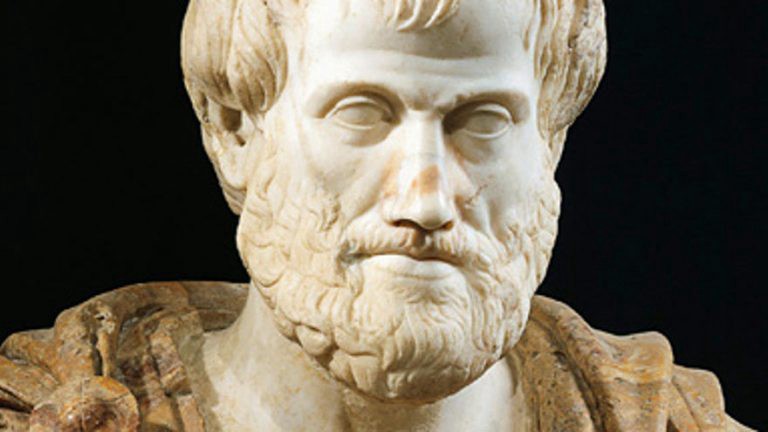
For example, most people value — at least to some degree — both privacy and security, but different people would make the trade-off between these values differently to get something else they want or need.
These value conflicts can directly impact the process of designing AI-based technologies. Some conflict examples include timeliness versus completeness (as when firm deadlines result in the release of low-quality products), innovation versus reliability (when leading-edge becomes bleeding-edge), and honesty versus obedience (because, when your boss says that they really want to know what you think, they don’t always mean it).
My goal is to get students to think about the ethical decisions involved in the design of AI-based technologies, not just the technical ones.
During the initial four-year phase of Good Systems, our focus will be on understanding how to define good systems, evaluate good systems, and build good systems. We must understand what a good system is. We must agree on ways to evaluate the goodness of a system. And we must apply these definitions and evaluations to building good systems.
In the second four years of Good Systems, we will focus on designing and implementing good systems, using and regulating good systems, and partnering with industry to build good systems with the aim of having a direct, beneficial impact on the world as a result of our research.
Ensuring that values drive the design of AI technologies is a grand challenge that will require collaboration among a wide range of experts, including humanists, social scientists, and technologists, as well as CEOs, government officials, and the everyday people who use AI technology.
Orchestrating such collaboration is difficult but important work, and our growing team of information and computer scientists, transportation and communication experts, ethicists and philosophers, engineers, librarians, and policy scholars (just to name a few) is up to the task. And in so doing, we’re taking a cue from our “colleague,” Dr. Ian Malcolm, whose articulation of this issue plainly sets the stakes for this challenge. Rather than seeing what we can do and what we should do as mutually exclusive, we are making it our core principle to ask both questions together in order to achieve the best possible outcome for society.