Overview
Estimating health outcomes at a neighborhood scale is important for promoting urban health, yet is costly and time-consuming. To help address this challenge, the Good Systems Urban Health Risk Mapping project developed an AI system that can measure the health effects of neighborhood environments using citizen crowd-sourced data. The research team mapped the computed health risk scores at the census tract-level for ten U.S. cities, including the City of Austin, and built an interactive website that allows users to understand how changes to their neighborhoods can affect their health. For example, if a user changes neighborhood sidewalk density, the website will show the expected changes in health behavior and outcomes, such as decreased physical activity level and increased diabetes risk.
Methods and Findings
The project presents a machine-learning-enabled approach to predicting the prevalence of six common non-communicable chronic diseases at the census tract level. Applying this approach to City of Austin data showed the method can yield fairly accurate predictions. In searching for the best predictive models, the research team experimented with eight different machine learning algorithms and 60 predictor variables that characterize the social environment, the physical environment, and the aspects and degrees of neighborhood disorder. Analysis suggests that the sociodemographic and socioeconomic variables are the strongest predictors for tract-level health outcomes, and the historical records of 311 service requests can be a useful complementary data source as the information distilled from the 311 data often helps improve the models' performance.
Applications
The machine learning models yielded from this study can help the public and city officials evaluate future scenarios and understand how changes in neighborhood conditions can lead to changes in the health outcomes. By analyzing where the most significant discrepancies between the predicted and the actual values are, areas of best practice and areas in need of greater investment or policy intervention can also be identified.
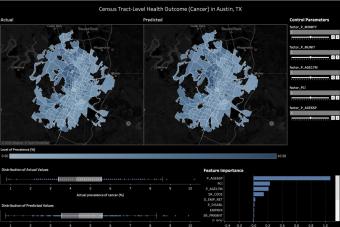

Urban Health Tracker
An interdisciplinary team of researchers developed an AI system that can measure the health effects of neighborhood environments using citizen crowd-sourced data.
Team Members
Valerie Danesh
Documents
Select Publications
Chen Feng and Junfeng Jiao. “Predicting and Mapping Neighborhood-Scale Health Outcomes: A Machine Learning Approach.” Computers, Environment and Urban Systems.
Chen Feng, Junfeng Jiao, and Hao Feng Wang. “Estimating e-scooter traffic flow using big data to support planning for micromobility.” Journal of Urban Technology.